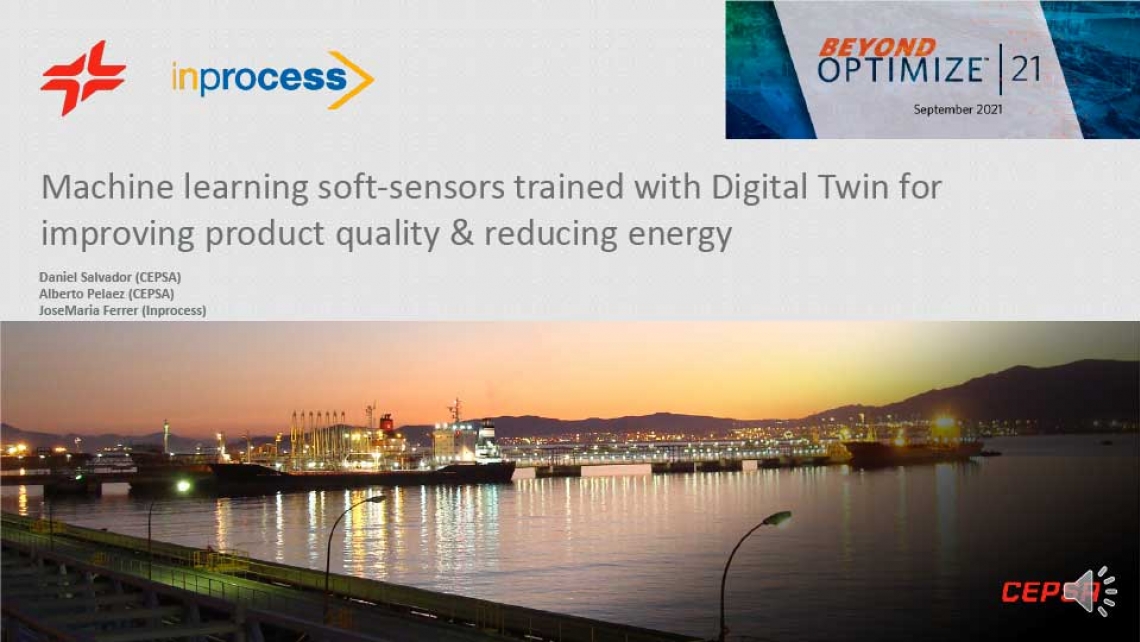
Inprocess participation in Beyond Optimize 2021: Machine learning soft-sensors trained with Digital Twin for improving product quality & reducing energy
Challenge
Cepsa implemented a new upgrade project to expand production at its Puente Mayorga Chemical Plant in San Roque (Cadiz). The revamping process covers the installation of the new Detal technology, co-licensed by Cepsa and UOP, the most modern and efficient technology for the production of linear alkylbenzene (LAB), as well as increasing production capacity at the plant from 200,000 to 250,000 tons.
CEPSA contracted Inprocess to develop a Digital Twin based on a rigorous dynamic process simulation model (HYSYS Dynamics) of the revamped plant, which is also connected to a detailed 3D Virtual Reality model of the plant. That Digital Twin is being used in a number of key project tasks including verify complex regeneration sequences, test and tune plant Operating Procedures, controllers pre-tuning, operator training with Emulated OTS and soft-sensors development.
Inprocess solution
Machine Learning is a branch of the Artificial Intelligence whose goal is to build systems that automatically learn from data and adjust actions or decisions accordingly.
Inprocess implemented the Digital Twin as required, but we put the strength in the re-use of the Digital Twin model for the specific purpose of the development of soft-sensors or inferentials that are currently being used to achieve better control of the quality and energy consumption of the considered columns.
The Aspen HYSYS models were intensively tested within all potential operating regimes (Feed composition, Column Pressure, Reboiler Duty, Reflux, etc), running multiple models in parallel to generate a large amount of process data for all the potential variables to be included in the soft-sensor.
Once the time series data was generated, it was used to develop a machine learning model for each soft-sensor and implement them in the plant as new process variables for the quality controllers.
Benefits
As a result, after the implementation of the Machine learning modeling for the soft sensors, benefits comprise, in general, improved product quality and reduced energy consumption, and in particular:
- 1.5% energy saving on each column involved.
- Quality and recovery improve on the top and bottom streams of the columns.
- Payback around 1 year or less. Minimum investment and good returns.
- Technically viable the data extraction from simulation to create and train machine learning models, even before plants start-up.
Such a contribution is already available for downloading from our website here.
For more information, please do not hesitate to get in contact with us.